Problem
The maritime industry's substantial greenhouse gas emissions, comprising around 2.8% of the global total, have prompted action. The International Maritime Organization (IMO) aims for net zero carbon emissions by 2050.
Efficiency. Insight. Action
At EnervAI, we use cutting-edge machine learning to revolutionize maritime operations. Our platform accurately predicts speed consumption and assesses hull and engine conditions, empowering users to optimize fuel usage and enhance sustainability efforts. Join us in shaping a sustainable future with EnervAI.
EnervAI
Sustainability made Affordable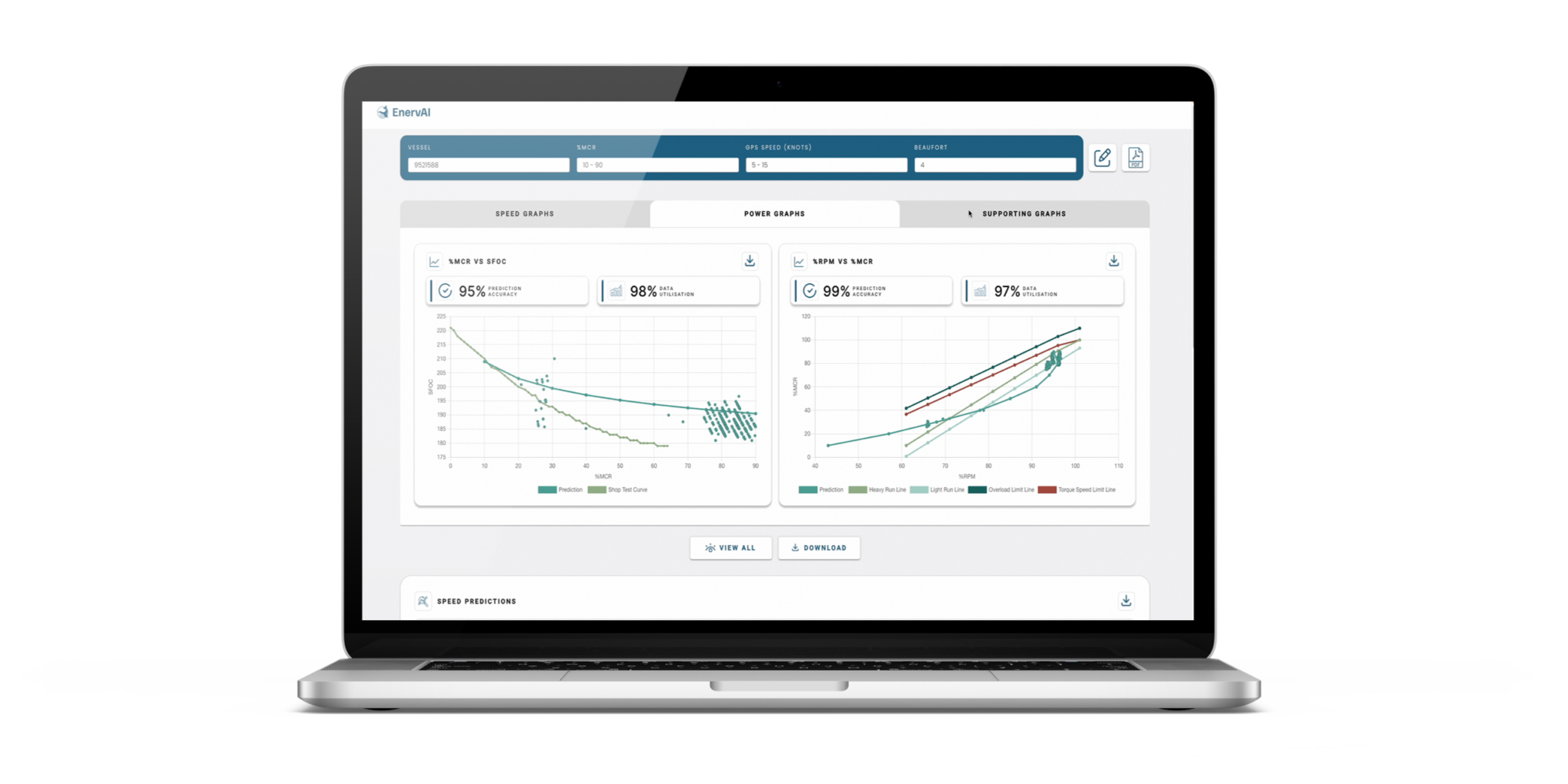
Design Journey
System Architecture
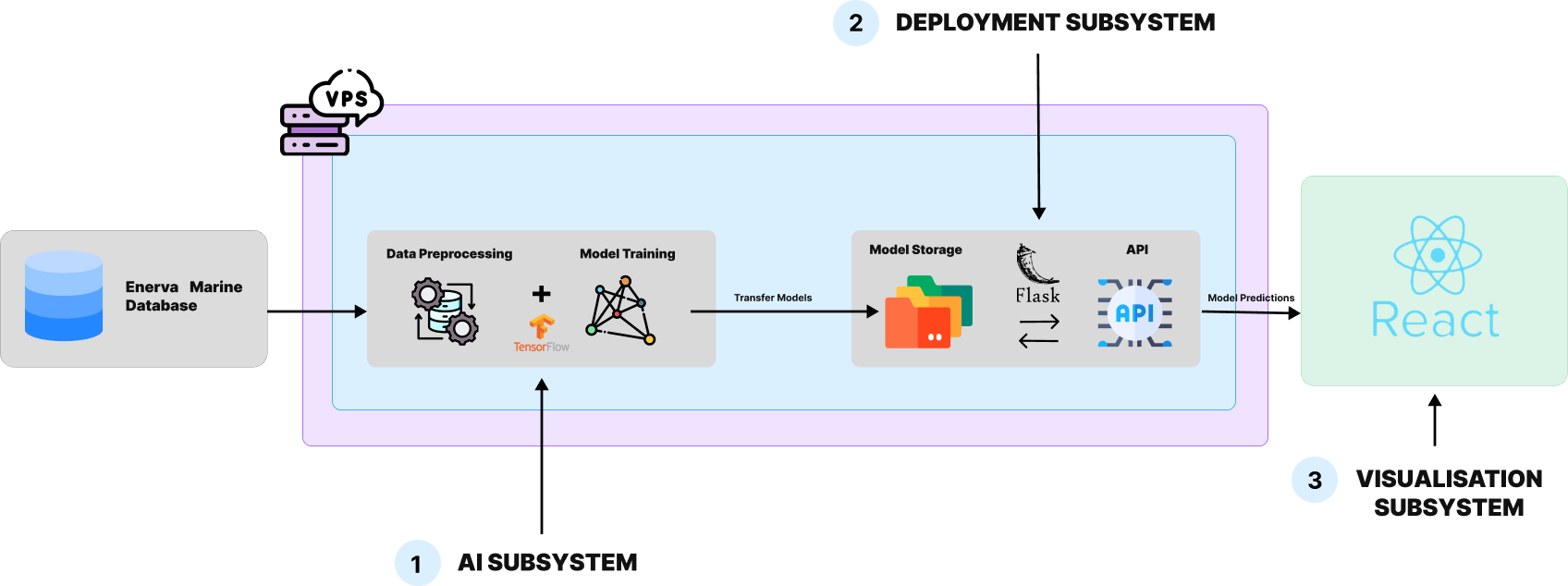
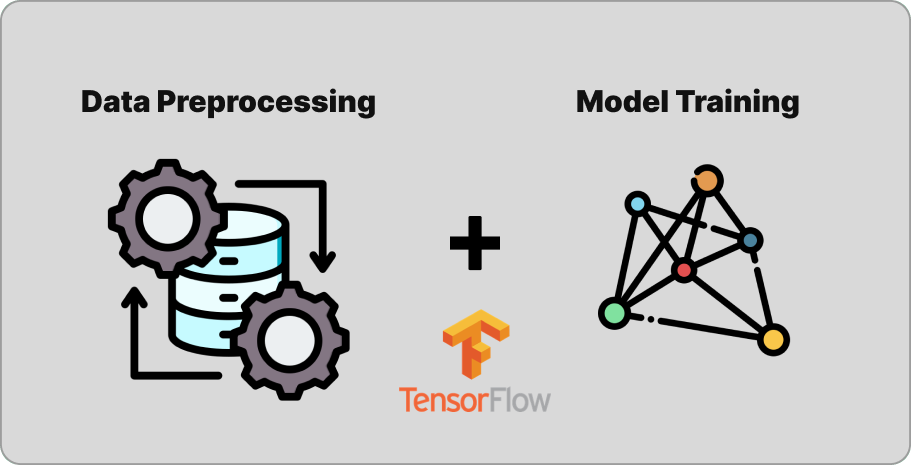
Model Training
We leveraged TensorFlow to train and customise deep learning models, utilizing high-frequency data extracted from Enerva Marine’s database. Prior to training, this data underwent preprocessing, ensuring it is optimally formatted and ready for input into our models.
Model Deployment
Upon the successful training of our deep learning models, they were seamlessly integrated into a server infrastructure, where models are securely stored and readily accessible. Through an API developed with Flask, web clients can make predictions with these models effortlessly.
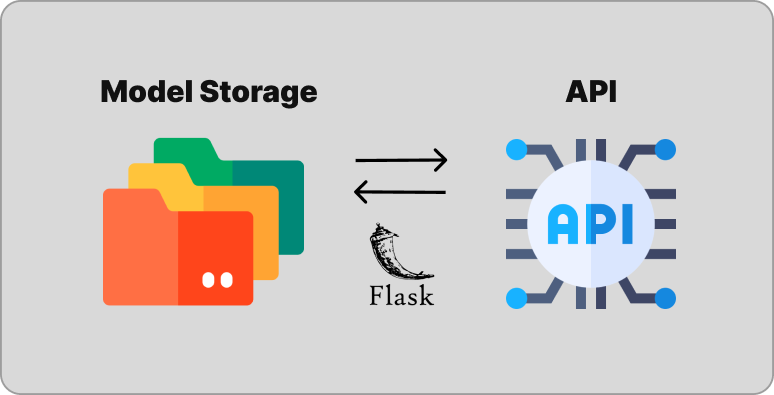
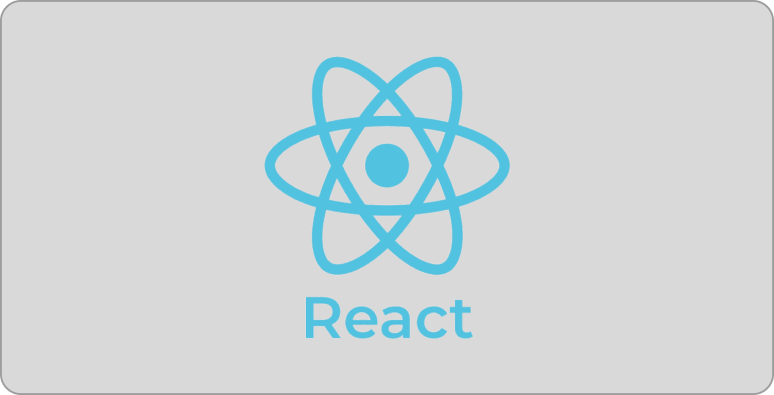
Model Visualisation
Utilising React, we rendered predictions into dynamic visualisations through intuitive graphs and detailed tables, serving as a powerful tool for visualising complex data. This enables stakeholders to make well-informed decisions based on the visualized predictions.
Project Achievements
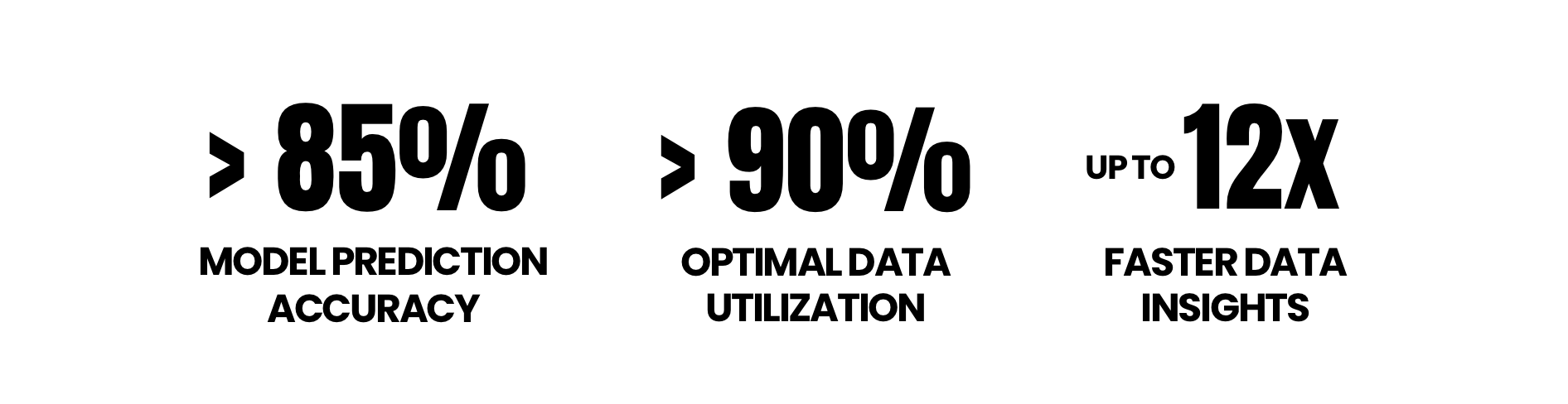
Acknowledgements
We sincerely thank our Capstone mentors, Dr. Francisco Benita and Dr. Thomas Schroepfer, for their invaluable guidance and support, shaping our project's success.
Our heartfelt gratitude goes to Dr. Susan Wong, our CWR mentor, whose insights enhanced the clarity and effectiveness of our project.
Special appreciation goes to Varisth Agarwal and Rajat Saxena, our company mentors, for their expert guidance throughout our project.
Finally, we appreciate the ongoing support from the SUTD Capstone Office, which was crucial to our project's progress.

References
Faber, J., Hanayama, S., Zhang, S., Pereda, P., Comer, B., Hauerhof, E., Schim Van Der Loeff, W., Smith, T., Zhang, Y., Kosaka, H., Adachi, M., Bonello, J.-M., Galbraith, C., Gong, Z., Hirata, K., Hummels, D., Kleijn, A., Lee, D. S., Liu, Y., . . . Xing, H. (2021). Fourth IMO GHG Study 2020. International Maritime Organisation. Retrieved November 24, 2023, from
https://wwwcdn.imo.org/localresources/en/OurWork/Environment/Documents/
Fourth%20IMO%20GHG%20Study%202020%20-%20Full%20report%20and%20annexes.pdf
Sidenvall Jegou, I., Laffineur, L., Spiegelenberg, F., & Madalena Leitao, A. (2023, September 22). Net-zero by 2050: Achieving shipping decarbonization through industry momentum and the new ambition at IMO. UNCTAD. https://unctad.org/news/transport-newsletter-article-no-108-net-zero-by-2050