Problem With Manual Report Writing
In military operations, timely and accurate intelligence reporting is critical for decision-making. However, the current manual report-filling process for documenting military observations and surveillance faces following challenges:
Limited Accuracy in Scene Understanding
Traditional surveillance and reconnaissance methods struggle with accurate object detection and scene captioning, making it difficult to extract meaningful intelligence from raw video data.
Cognitive Overload on Soldiers
Manually analyzing combat scenes and writing reports under high-stress conditions burdens soldiers, leading to fatigue, slower responses, and reduced mission effectiveness.
Delayed Decision-Making
Military operations require quick decision-making, but existing systems do not provide automated, real-time insights from combat footage.
Human Error & Inconsistency
Current battlefield reporting relies heavily on manual documentation, which is slow, inefficient, and prone to human errors.
Introducing,
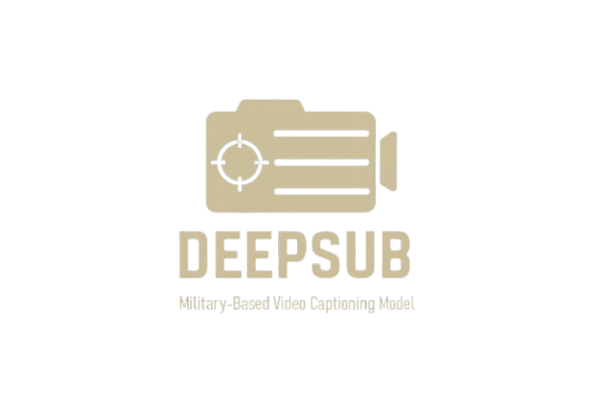
Transforming Battlefield Footage into Actionable Intelligence.
Our Solution
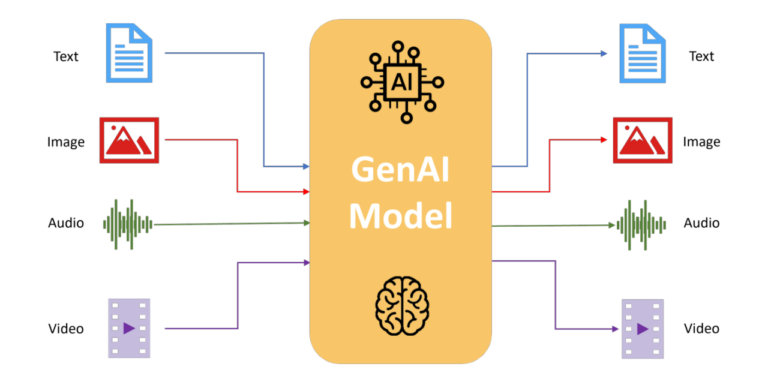
What is a Multimodal Model?
InternVL is a powerful multimodal model that processes both visual and textual inputs, enabling deeper scene understanding than traditional image captioning models. Unlike single-modality systems that rely solely on images or text, multimodal models like InternVL can reason across both, making them more effective for complex tasks like visual question answering and context-aware image captioning.
InternVL: Bridging Vision and Language
InternVL is a cutting-edge multimodal model that seamlessly integrates visual and textual data, enabling deep comprehension of scenes and their contextual meanings. It processes an image or video frame alongside textual prompts to produce accurate, descriptive, and context-aware outputs. InternVL stands out due to its large-scale pretraining across diverse datasets and its ability to handle open-domain and task-specific challenges effectively.
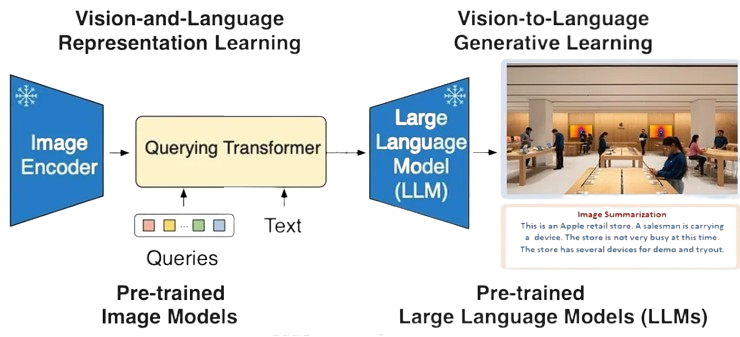
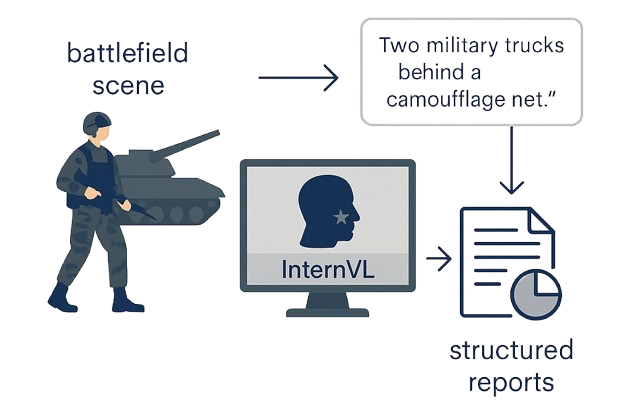
How We Use InternVL in Our Project
In our project, InternVL is the core engine for battlefield scene interpretation. We fine-tuned it on a curated military dataset to help the model specialize in detecting and understanding combat-related elements—like military vehicles, personnel, weapons, and formations. By feeding each frame of a video into InternVL, the model generates precise and context-sensitive captions. These captions are then compiled into structured reports, automating the task of analyzing combat footage and enabling quicker, data-driven decision-making in mission-critical environments.
Finetuning Workflow
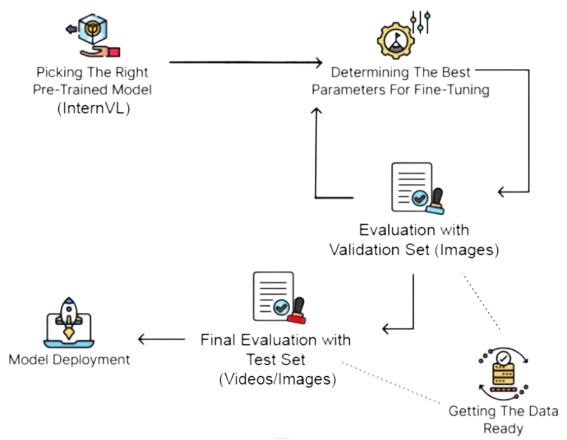
Hardware Used
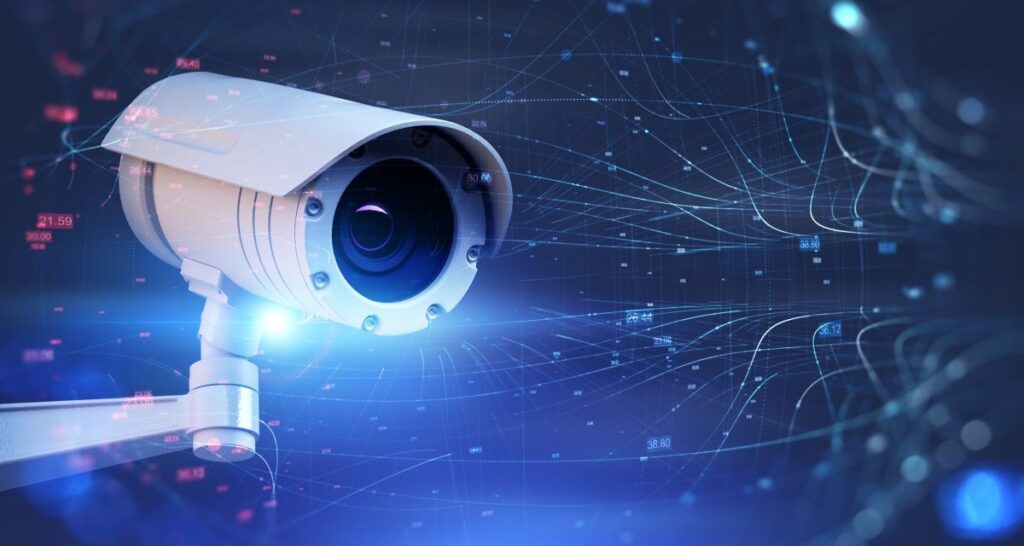
Camera for Real-Time Video Capture
A battlefield-mounted camera captures live video streams, providing real-time input to the model for scene analysis and automated reporting.
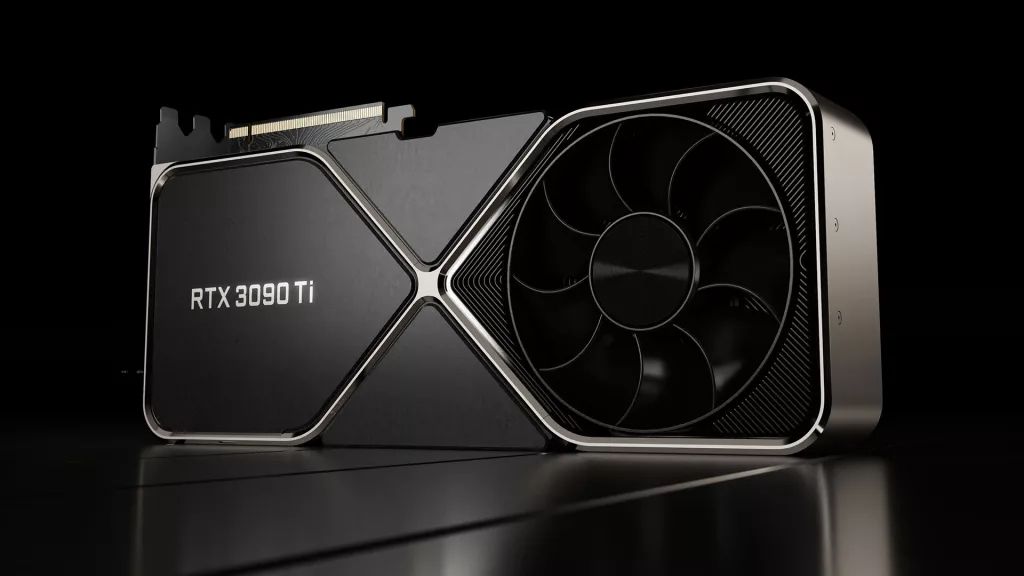
GPU for Model Training and Inference
A GPU accelerates both model training and real-time inference, enabling fast, accurate processing of video feeds for instant scene understanding.
Tech Stack & Platforms Used
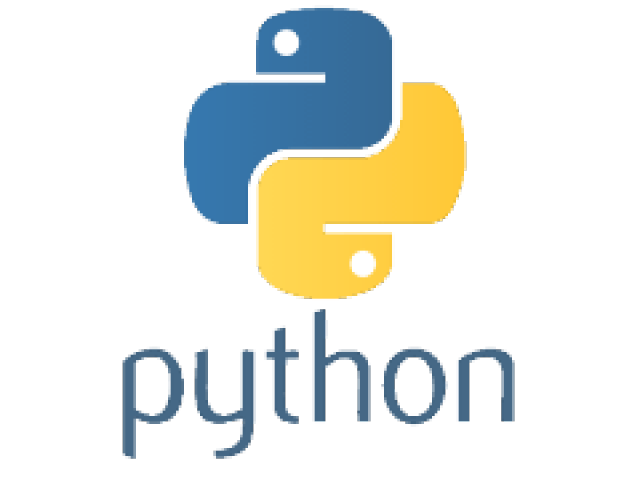
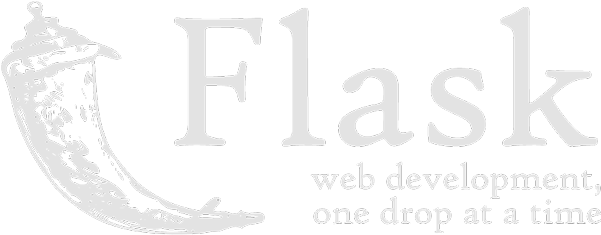
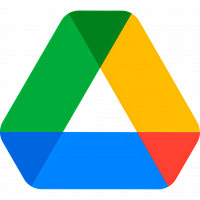
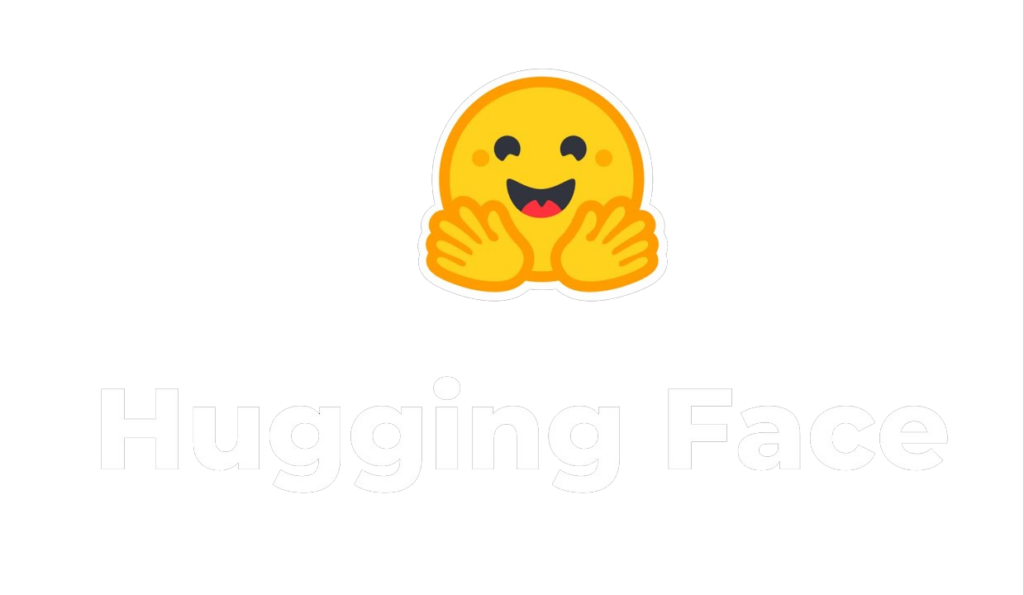
Project Video
Live Captioning Demo
Evaluation/Results
To assess the performance of our system, we evaluated the captioning quality using standard Natural Language Generation (NLG) metrics such as BLEU, METEOR, and CIDEr. These metrics compare the generated captions against human-annotated references to measure precision, relevance, and fluency.
User Testimonial
After using DeepSub, “…we believe this will significantly improve report accuracy while reducing the cognitive load on soldiers, resulting in a strategic advantage during missions. As the industry mentor, I am proud of the team’s effort and determination to see the success of this project.”
– Mr. Hing Wen Siang, Army Division Lead, AI Dev, ST Engg.
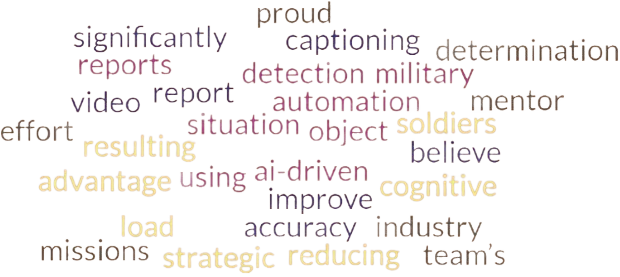
Our Team
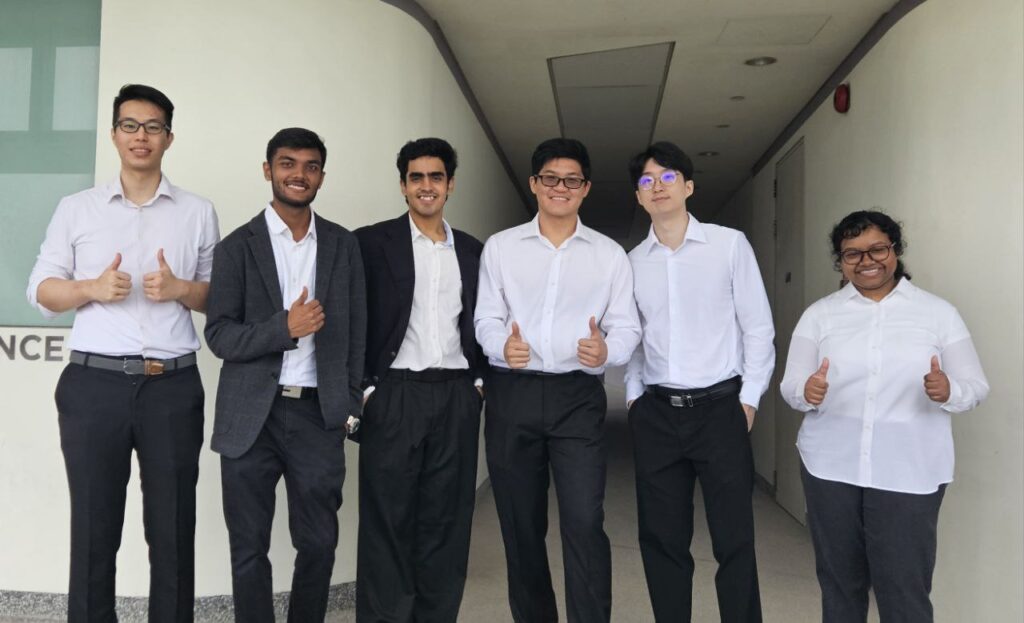
Acknowledgements
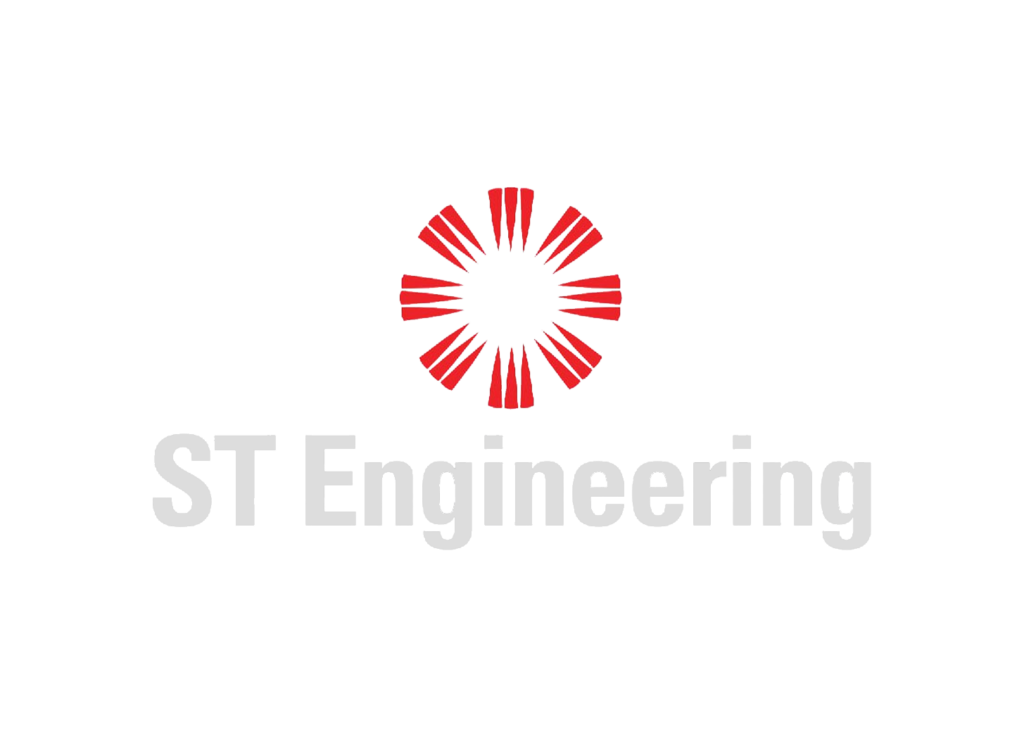